Within the rapidly shifting world of artificial intelligence, generative AI shines as a key player for the next wave of online content making. In fact, forecasts suggest that the value of the generative AI sector could hit $66.62 billion in 2024. Whether it’s producing lifelike visuals or crafting text that mimics human writing, the potential of AI to innovate and produce seems limitless.
Yet, the real power source for such sophisticated AI functions rests in the technologies that allow computers to comprehend, analyze, and create intricate data. Vector databases stand out among these technologies, serving as an essential tool for storing and handling the complex, multi-dimensional data that AI algorithms depend on.
But what is a vector database exactly and how it can help fuel generative AI tools? In this post, we’ll delve into the crucial function of vector databases, showcasing how they contribute to the more efficient and effective creation of AI-generated content.
What is a Vector Database?
At the heart of numerous AI applications lies the notion of vector databases, a specialized database type crafted to handle high-dimensional vector data. Unlike conventional databases that organize data in rows and columns, vector databases are tailored to oversee data presented as vectors—a format that translates information about text, images, and sounds into numerical values interpretable by AI models.
This distinct capability positions vector databases as indispensable tools for AI, enabling the efficient storage, retrieval, and manipulation of intricate data. Key functionalities like similarity search, which identifies vectors close to a given query vector, and scalable indexing mechanisms, ensure that vector databases can meet the demanding needs of generative AI models.
The Impact of Vector Databases on Generative AI
The significance of vector databases in generative AI lies in the role of swiftly retrieving pertinent data points required for content creation. In tasks like generating images, producing language, and crafting personalized content, the capability to promptly locate and employ comparable data points holds immense importance.
For example, when a generative AI system creates a new image, it needs to sift through extensive datasets to locate images sharing similar characteristics. Vector databases simplify this task by facilitating rapid searches for similarities, thereby enhancing the efficiency and quality of the model’s output. This efficiency is critical for delivering immediate responses in AI-powered applications, ensuring that generative models can create high-quality, contextually appropriate content on a large scale.
Advantages of Using Vector Databases in AI Projects
Implementing vector databases in AI projects offers numerous advantages, particularly in terms of speed, accuracy, and flexibility. When dealing with large datasets, which are typical in AI training and deployment, vector databases significantly enhance the speed and efficiency of data handling.
This improvement is crucial for scalable AI models that require fast access to vast amounts of data. Additionally, the accuracy of AI-generated content benefits from the high-quality data management provided by vector databases. By maintaining the integrity of complex data in a form that AI models can easily process, these databases ensure that the outputs are as precise and relevant as possible.
Additionally, the flexibility of vector databases accommodates various data types and uses. Whether it’s analyzing human language intricacies in chatbots or capturing detailed artwork elements, vector databases provide an adaptable framework suitable for diverse generative AI tasks. This flexibility is crucial for delving into uncharted territories in AI-based content creation, empowering developers and researchers to expand the limits of what can be achieved.
Challenges and Considerations
Even though there are undeniable advantages, adopting vector databases for AI projects brings its own set of difficulties and points to ponder. Technical barriers, like the intricacies involved in establishing and upkeeping these databases, can be daunting challenges, particularly for entities lacking deep knowledge in managing databases.
Moreover, as AI applications weave their way deeper into everyday scenarios, issues related to data privacy and safeguarding become more critical. It’s essential to ensure that personal and sensitive data within vector databases are shielded, necessitating strong security protocols and responsible handling of data.
On top of that, the swift advancement in AI technology calls for ongoing refinement and updates to vector database technologies. Keeping in step with the changing demands of AI models requires developers to be alert and proactive, ready to adopt the latest in database management and data processing techniques to keep AI applications sharp and effective.
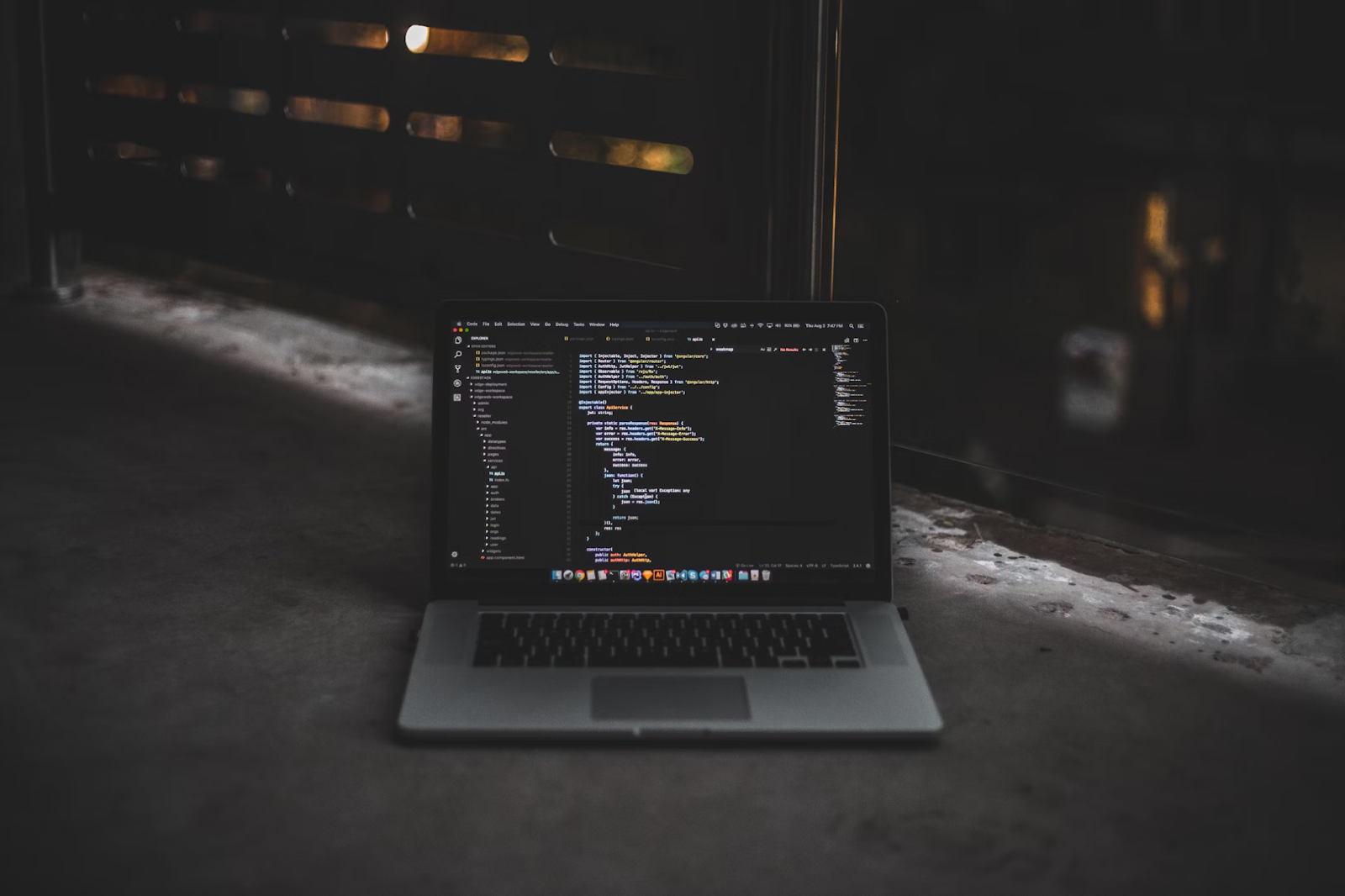
Final Thoughts
Vector databases are the backbone of blending data management with the tech of artificial intelligence, serving as pivotal support for the next leaps in generative AI innovations. They’re essential for managing intricate data smoothly, thereby boosting AI models’ ability to craft, enhance, and tailor content uniquely. With the AI landscape expanding rapidly, the significance of vector databases will only climb, underlining the urgency for continuous exploration and enhancement in this domain.
Looking ahead, the evolution of generative AI powered by vector databases heralds an era where AI-crafted content becomes even more lively, customized, and significant. As we dive deeper into this thrilling phase of tech progress, the refinement and advancement of vector databases will surely open doors to new horizons, steering the course of artificial intelligence into territories yet to be fully envisioned.